“Garbage in, garbage out” (GIGO) means if garbage is input into a computer, it will then deliver poor output. The phrase is common in the world of computer science and data analytics and reflects the problem that many organizations are facing. The problem results from the use of the data that lack quality, accuracy and precision and leads to wrong business decisions. This can happen even if the data undergo advanced analyses with artificial intelligence (AI) or machine learning (ML).
AI and ML are the technologies that many business organizations want to use to enhance their competitive edge. In fact, the use of AI and data analytics for advanced data analyses is a latter stage in data use. Most organizations focus on this part and overlook the important stage of having data architecture to possess strong bases and valuable data. If unverified or substandard data are processed or analyzed with AI, results may be inaccurate or even wrong and lead to the business decisions that may cause unexpected loss or damage in the future.
Bluebik would like to show how data architecture can give the quality data that will unlock AI potential and turn businesses into data-driven organizations as expected.
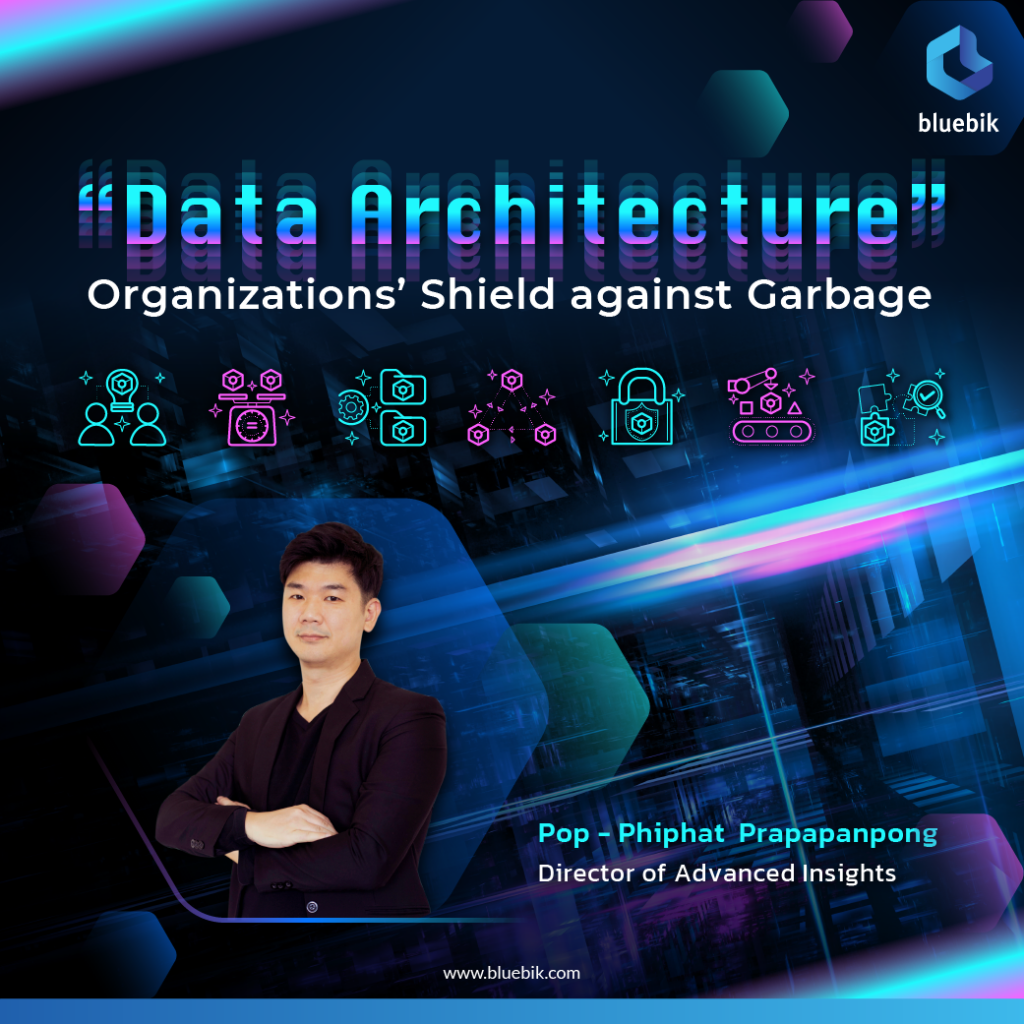
What is data architecture?
Data architecture is like a blueprint for the direction, data quality, and other factors that will guarantee efficient and organized data management. It also makes data use agile and flexible in the way that business requirements will be fulfilled accurately and precisely. The important elements of data architecture are as follows.
1. Data sources – Organizations have various sources of data and their data are in many forms including structured data, semi-structured data and unstructured data such as data from databases, data from applications and pictures.
2. Data modeling – It refers to the design of data models to satisfy business requirements.
3. Data integration – It concerns channeling raw data from their sources to data lakes and data warehouses.
4. Data pipeline and data flow – This is to determine the natures of input – batches or real-time input, regulate input and transform input into the designed data models.
5. Data storage – Data can be stored in the data lakes that are the sources of raw data, the data warehouses which keep the data that passed a data cleansing process and are readied for analyses or the data marts which gather data for presentations, reports and executives’ dashboards.
6. Data serving – It refers to the channels that offer ready-to-use data to organizations. The channels can be databases and application programming interface (API), among others.
The development of data architecture is all for the “data of truth”
1. Business understanding – It will lead to the data modeling which creates the attributes of data for data categorization. It will also identify the data that meet the demand of organizations.
2. Data storage standard – It facilitates data use and data integration and supports future systems.
3. Methodology for data storage and operation – It sets the data sources that will be the cores of data models and determines the flow of data to be processed. There must be a clear picture of this process and the clear components of data management consisting of data storage places, the technologies that are applied and the sources of data to be processed.
4. Data connection and exchange – Technological application is designed to support the connection and exchange of data among many data sources which may require different methods of connection. Some applications may require data connection through API and other applications can connect directly to databases.
5. Data security – Authority to access data is set with data classification. There are public data, the internal data of individual business units and exclusive data for executives. The design of data access authority is aimed at offering basic data security to organizations.
6. Data categorization – There are master data and reference data. With data categorization, data can be acquired more accurately and precisely. For example, without reference data, a company that needs the exchange rates that are always changing may have different rates among business units because the rates come from different sources.
7. Data cleansing – It guarantees the quality of data. However, data cleansing cannot be done for all attributes of data. Therefore, data architects together with users must set critical data elements (CDE) to specify the data that are important and need absolute accuracy for the sake of advanced analyses.
8. Design of data acquisition for analyses – This is another important step towards the creation of data architecture. The acquisition of stored data must be flexible and ensure that subsequent data analyses will be convenient and smooth.
Professionally designed data architecture means organizations’ advantage and reduced risks
Good data architecture is not just a framework of the best practices for organizations. Its design requires understanding of both business and technology. The vendors that mainly have technology expertise may design the data models that serve the demand of industries. However, data modeling has a frequent problem. Some necessary attributes do not exist in common models. This results from the lack of knowledge and understanding of business. Therefore, the design of the architecture that truly supports data analyses, reduces human errors and cuts operating costs and workers’ hours need the experienced designers who have thorough business knowledge and technological expertise to eliminate risks.
It is now undeniable that data are the heart of business. Previously used instinct and experience may compromise the growth of organizations in the future especially when competitors become data-driven organizations. The first organizations that can handle data professionally with data architecture have their competitive edge in the era of accelerating change.
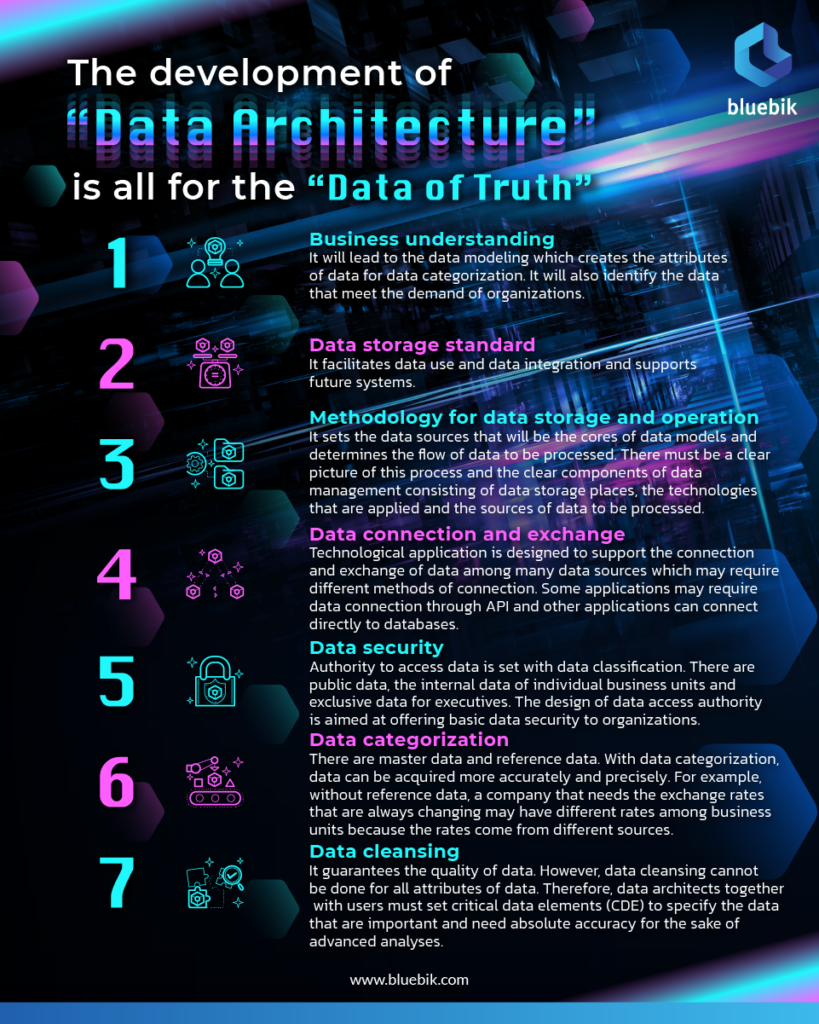